Contents
- 1 The Role of Machine Learning in Enhancing Compromise Assessments
- 1.1 1. Understanding Machine Learning in Cybersecurity
- 1.2 2. Enhancing Threat Detection with Machine Learning
- 1.3 3. Improving Incident Response with Machine Learning
- 1.4 4. Adapting to New Threats with Machine Learning
- 1.5 5. Real-World Applications of Machine Learning in Compromise Assessments
- 1.6 6. Future Trends in Machine Learning for Compromise Assessments
- 1.7 Conclusion
- 1.8 CTA
- 1.9 Elevate Your Cyber security with SentryCA
The Role of Machine Learning in Enhancing Compromise Assessments
In the rapidly evolving world of cybersecurity, traditional methods of compromise assessment are no longer sufficient to combat the sophisticated threats of today. The Role of Machine Learning in Enhancing Compromise Assessments has become increasingly significant as organizations seek advanced ways to stay ahead of attackers. Enter machine learning (ML), a transformative technology that is revolutionizing how organizations detect, analyze, and respond to security threats. This blog post explores how machine learning enhances compromise assessments, provides real-world applications, and discusses future trends in this critical field.
1. Understanding Machine Learning in Cybersecurity
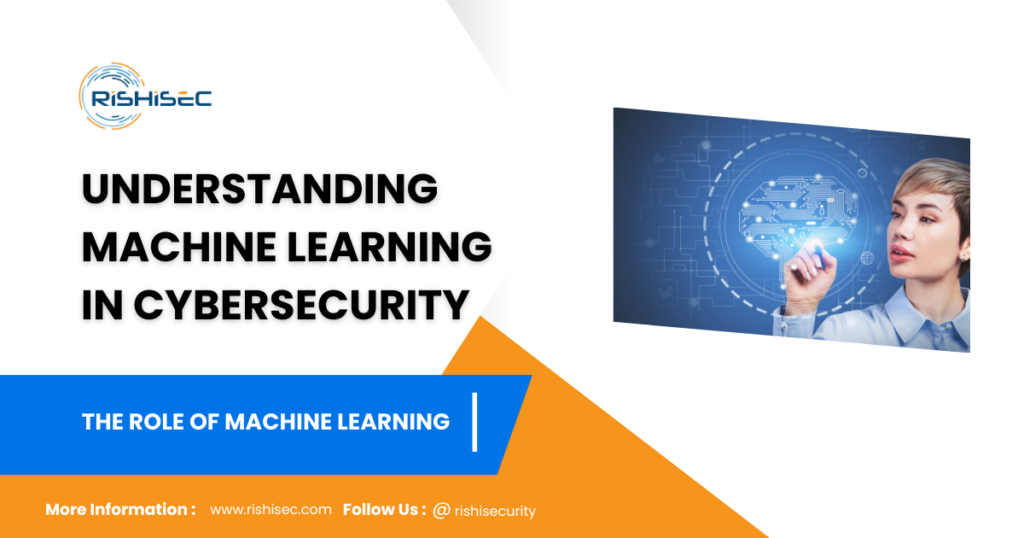
Machine Learning in Cybersecurity
Machine learning is a subset of artificial intelligence that involves training algorithms to recognize patterns and make decisions based on data. In contrast to traditional software that follows explicit instructions, ML systems learn from data and improve over time. Moreover, in cybersecurity, ML algorithms can analyze vast amounts of data so that they detect anomalies and predict potential threats more effectively.
How Machine Learning Works
At its core, machine learning uses data to train models that can identify patterns. In cybersecurity, these models are trained on data sets containing both normal and malicious activity. For example, supervised learning models are trained with labeled data, where the types of attacks are known, while unsupervised learning models work with unlabeled data, identifying new and unknown threats by detecting deviations from normal behavior.
2. Enhancing Threat Detection with Machine Learning
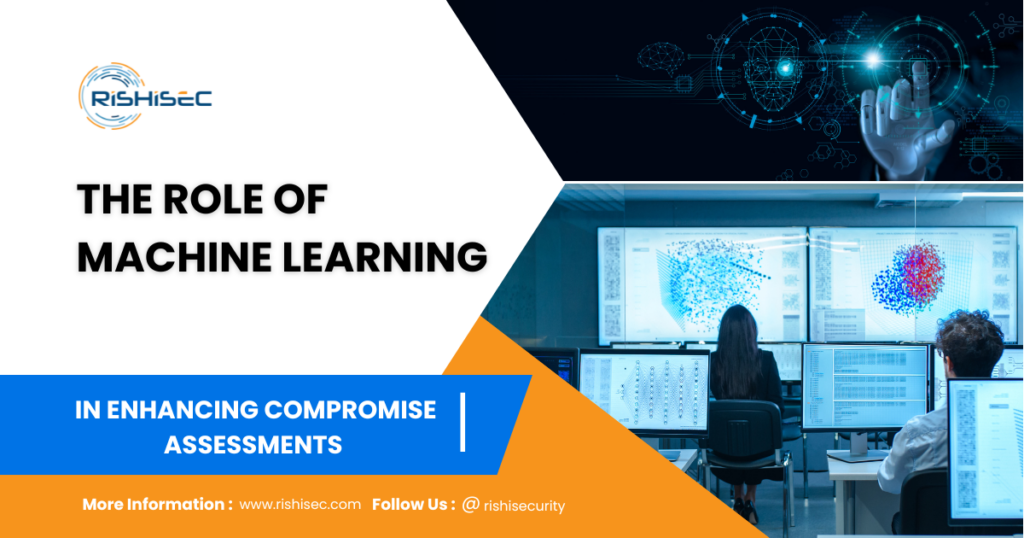
Threat Detection with Machine Learning
One of the most significant advantages of machine learning in compromise assessments is its ability to enhance threat detection. ML algorithms can process and analyze large volumes of data in real-time, identifying potential threats more quickly and accurately than traditional methods.
Anomaly Detection
Machine learning excels at anomaly detection, which involves identifying deviations from established patterns. For instance, if a network experiences a sudden spike in traffic or an unusual login pattern, ML algorithms can flag these anomalies as potential security threats. This capability is particularly valuable for detecting novel or unknown threats that do not match known attack signatures.
Predictive Analytics
Predictive analytics is another powerful application of machine learning. By analyzing historical data, ML models can forecast potential future threats and vulnerabilities. This proactive approach allows organizations to address potential issues before they escalate into actual breaches.
3. Improving Incident Response with Machine Learning
In addition to detecting threats, machine learning can significantly improve incident response. Automated systems powered by ML can prioritize alerts based on the severity and potential impact of the threat, allowing cybersecurity teams to focus on the most critical issues.
Automated Response
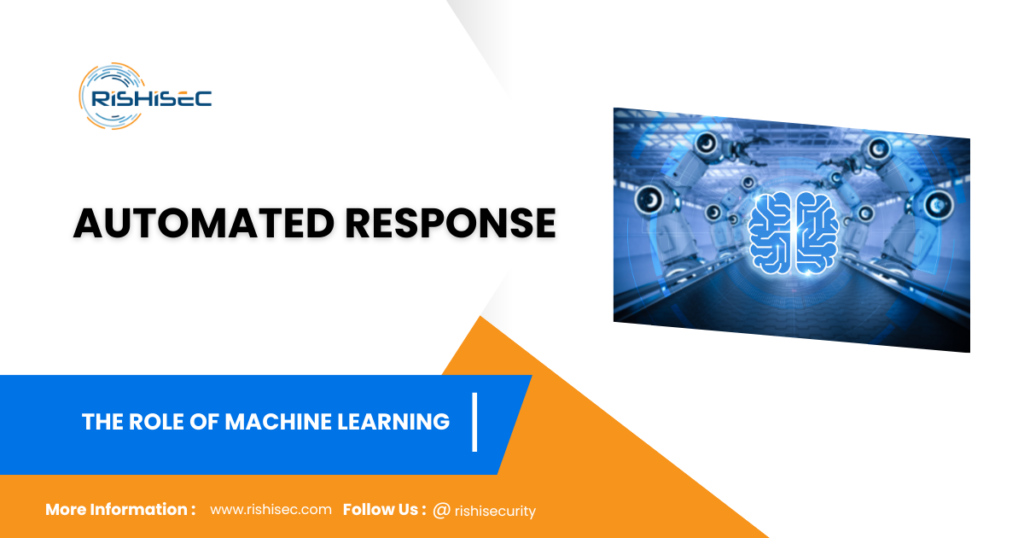
Machine learning can also automate response actions, reducing the time it takes to mitigate threats. For example, if an ML system detects a potential breach, it can automatically isolate affected systems, block malicious IP addresses, or initiate other predefined response actions. This automation helps minimize damage and reduce the workload on security teams.
Enhanced Decision-Making
Machine learning-driven analytics provide valuable insights that enhance decision-making during an incident. By analyzing the context and impact of a threat, ML systems can offer recommendations on the best course of action, improving the overall effectiveness of the response.
4. Adapting to New Threats with Machine Learning
One of the key benefits of machine learning is its ability to adapt to new and evolving threats. As cyber threats continue to evolve, ML algorithms can continuously learn from new data and update their detection models accordingly.
Continuous Learning
Machine learning models improve over time as they are exposed to more data, so they become more effective at detecting evolving threats. This continuous learning process not only allows ML systems to stay current with the latest threat trends, but also enables them to adapt to new attack techniques seamlessly. For example, if a new type of malware emerges, ML models can quickly learn to recognize its signatures and behaviors, and provide timely protection against emerging threats.
Integration with Other Security Tools
Machine learning can be integrated with other security tools and systems so that it provides a comprehensive security solution. For example, not only can ML enhance endpoint protection, but it can also improve network monitoring and threat intelligence platforms, thus creating a multi-layered defense strategy that leverages the strengths of various technologies. In addition, this integrated approach ensures better visibility and faster response to potential threats.
5. Real-World Applications of Machine Learning in Compromise Assessments
Machine learning is already making a significant impact across various industries. Here are some real-world applications:
Financial Sector
In the financial industry, machine learning is used to detect fraudulent transactions and prevent financial crimes. ML algorithms analyze transaction patterns to identify suspicious activities, such as unusual spending or account access from unfamiliar locations.
Healthcare Sector
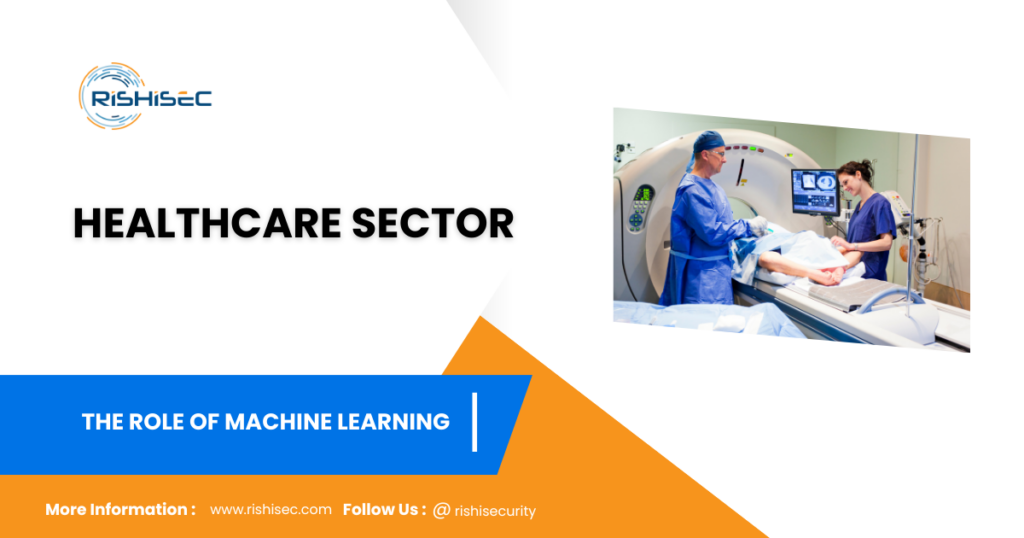
Healthcare organizations use machine learning to protect sensitive patient data from cyberattacks. ML models analyze access patterns and detect anomalies that could indicate a data breach or unauthorized access.
Government and Defense
Government agencies and defense organizations leverage machine learning for national security purposes. ML systems analyze large volumes of data to detect potential threats, such as cyber-espionage or terrorist activities.
6. Future Trends in Machine Learning for Compromise Assessments
As machine learning technology continues to advance, its role in cyber security will evolve as well. Here are some future trends to watch:
Advanced Algorithms
Future developments may include more sophisticated algorithms that improve detection accuracy and reduce false positives. Research into new machine learning techniques, such as deep learning and reinforcement learning, could lead to breakthroughs in threat detection and response.
Enhanced Integration
Machine learning will increasingly be integrated with other emerging technologies, such as blockchain and quantum computing, to create more robust security solutions. This integration will enhance the ability to detect and respond to complex and multi-faceted threats.
Greater Automation
The automation of security processes will continue to grow, with machine learning driving more automated threat detection, response, and remediation. This trend will help organizations respond to threats faster and more effectively, reducing the risk of security breaches.
Conclusion
Machine learning is transforming the field of compromise assessments by providing more accurate, adaptive, and efficient threat detection and response capabilities. As organizations face increasingly sophisticated cyber threats, integrating ML into their cybersecurity strategies not only enhances defense but also becomes essential for staying ahead of potential attacks. Therefore, adopting ML technologies will play a critical role in maintaining a proactive security posture.
CTA
Elevate Your Cyber security with SentryCA
Don’t wait for a security breach to disrupt your operations. Leverage the power of machine learning with SentryCA’s advanced solutions for compromise assessments. Our state-of-the-art technology offers real-time threat detection and adaptive security measures tailored to your industry’s specific needs.
Experience the future of cyber security today. Sign up for a free trial of SentryCA and discover how our solutions can enhance your security posture and safeguard your organization against emerging threats.
Continue your cyber security journey while reading this post, The Future of Compromise Assessments in Cybersecurity